Artificial intelligence (AI) is making waves in the medical world, and its latest breakthrough is in diagnosing adolescent idiopathic scoliosis (AIS). Traditionally reliant on manual radiographic measurements, scoliosis assessments are now getting a high-tech upgrade with deep learning models that promise greater accuracy, speed, and consistency. Let’s dive into how AI is reshaping the future of scoliosis diagnosis and treatment.
The Challenge of Accurate AIS Assessment
AIS is a common spinal disorder affecting adolescents, often requiring precise evaluation to determine severity and treatment options. The gold standard for scoliosis assessment has been measuring the Cobb angle (CA) using radiographic images, with a CA greater than 10° confirming a scoliosis diagnosis. However, manual measurement methods are prone to variability among radiologists and can be time-consuming. In a busy clinical setting, this can slow down diagnosis and impact treatment planning.
Deep Learning to the Rescue
To overcome these challenges, researchers have developed AI-driven deep learning models to automate scoliosis assessment with high precision. One recent study introduced a ResNet34-based deep learning model enhanced with U-net characteristics. Trained on 600 full spinal radiographs, this model automatically identifies key anatomical landmarks and calculates spinal alignment parameters, streamlining the diagnostic process.
Meanwhile, another study utilized an RTMpose deep learning model to measure sagittal plane parameters in AIS patients. The model demonstrated high consistency with expert radiologists, proving that AI can enhance efficiency without sacrificing accuracy.
Promising Outcomes
The results speak for themselves. The ResNet34-based model achieved a mean absolute difference (MAD) of 2.15° for coronal angles and 2.72° for sagittal angles, with intraclass correlation coefficients (ICC) of 0.985 and 0.927, respectively. The RTMpose model, on the other hand, exhibited ICC values ranging from 0.892 to 0.991, aligning closely with the assessments made by experienced physicians.
These automated approaches not only reduce measurement variability but also allow for more efficient scoliosis monitoring over time. By integrating AI into clinical workflows, doctors can improve diagnosis speed and reliability while freeing up time for patient care.
The Future of AI in Scoliosis Care
As AI continues to evolve, its role in scoliosis assessment is expected to expand. Future developments may include real-time AI-assisted diagnosis, predictive analytics for scoliosis progression, and AI-driven treatment planning. With ongoing research and refinement, these technologies could transform how scoliosis is managed, offering a new level of precision and accessibility in patient care.
AI is proving to be more than just a trend—it’s a game-changer for scoliosis diagnosis and beyond. Stay tuned, because the future of AI in scoliosis care is just getting started!
References
- “Deep learning model for automatic radiographic measurement of adolescent idiopathic scoliosis parameters,” Journal of Orthopaedic Surgery and Research. Link
- “RTMpose deep learning model for automated sagittal plane parameter measurement in adolescent idiopathic scoliosis,” Journal of Orthopaedic Surgery and Research. Link
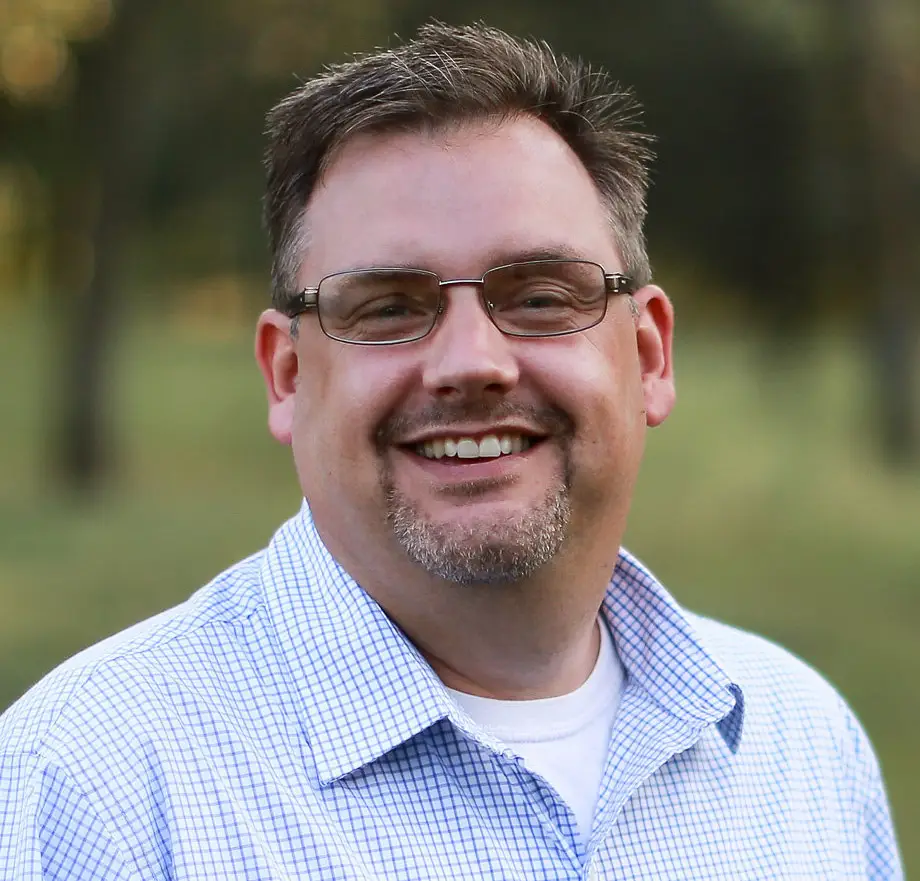